Chatbot Hallucinations Increase with Short Prompts: Study
A recent study reveals a concerning trend: chatbots are more prone to generating nonsensical or factually incorrect responses—also known as hallucinations—when you ask them for short, concise answers. This finding has significant implications for how we interact with and rely on AI-powered conversational agents.
Why Short Answers Trigger Hallucinations
The study suggests that when chatbots receive short, direct prompts, they may lack sufficient context to formulate accurate responses. This can lead them to fill in the gaps with fabricated or irrelevant information. Think of it like asking a person a question with only a few words – they might misunderstand and give you the wrong answer!
Examples of Hallucinations
- Generating fake citations or sources.
- Providing inaccurate or outdated information.
- Making up plausible-sounding but completely false statements.
How to Minimize Hallucinations
While you can’t completely eliminate the risk of hallucinations, here are some strategies to reduce their occurrence:
- Provide detailed prompts: Give the chatbot as much context as possible. The more information you provide, the better it can understand your request.
- Ask for explanations: Instead of just asking for the answer, ask the chatbot to explain its reasoning. This can help you identify potential inaccuracies.
- Verify the information: Always double-check the chatbot‘s responses with reliable sources. Don’t blindly trust everything it tells you.
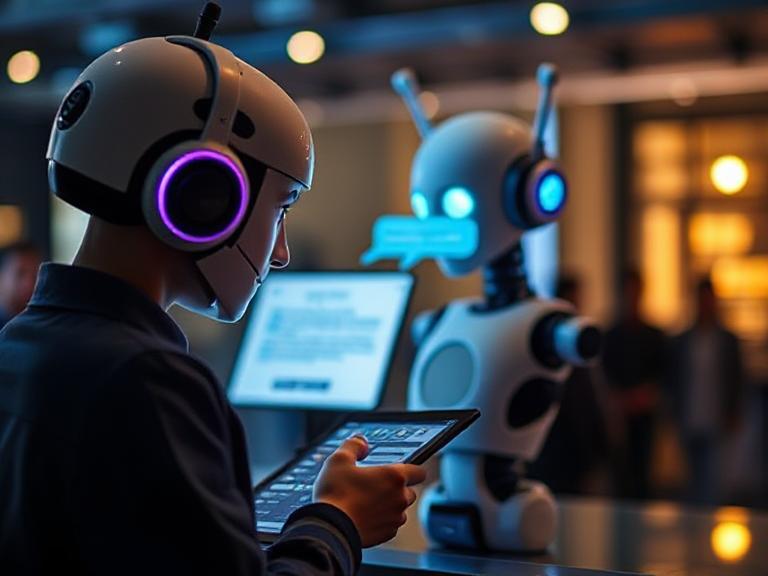
Implications for AI Use
You’re absolutely right to emphasize the importance of critical thinking and fact-checking when using AI chatbots. While these tools can be incredibly helpful, they are not infallible and can sometimes provide misleading information. As AI technology advances, understanding its limitations and using it responsibly becomes increasingly crucial.
🧠 Understanding AI Hallucinations
AI hallucinations occur when models generate content that appears plausible but is factually incorrect or entirely fabricated. This issue arises due to various factors, including:
- Training Data Limitations: AI models are trained on vast datasets that may contain inaccuracies or biases.
- Ambiguous Prompts: Vague or unclear user inputs can lead to unpredictable outputs.
- Overgeneralization: Models may make broad assumptions that don’t hold true in specific contexts.
These hallucinations can have serious implications, especially in sensitive fields like healthcare, law, and finance.
🔧 Techniques for Reducing AI Hallucinations
Developers and researchers are actively working on methods to mitigate hallucinations in AI models:
1. Feedback Loops
Implementing feedback mechanisms allows models to learn from their mistakes. Techniques like Reinforcement Learning from Human Feedback (RLHF) involve training models based on human evaluations of their outputs, guiding them toward more accurate responses.
2. Diverse and High-Quality Training Data
Ensuring that AI models are trained on diverse and high-quality datasets helps reduce biases and inaccuracies. Incorporating varied sources of information enables models to have a more comprehensive understanding of different topics.
3. Retrieval-Augmented Generation (RAG)
RAG involves supplementing AI models with external knowledge bases during response generation. By retrieving relevant information in real-time, models can provide more accurate and contextually appropriate answers.
4. Semantic Entropy Analysis
Researchers have developed algorithms that assess the consistency of AI-generated responses by measuring “semantic entropy.” This approach helps identify and filter out hallucinated content.
🛠️ Tools for Fact-Checking AI Outputs
Several tools have been developed to assist users in verifying the accuracy of AI-generated content:
1. Perplexity AI on WhatsApp
Perplexity AI offers a WhatsApp integration that allows users to fact-check messages in real-time. By forwarding a message to their service, users receive a factual response supported by credible sources.
2. Factiverse AI Editor
Factiverse provides an AI editor that automates fact-checking for text generated by AI models. It cross-references content with reliable sources like Google, Bing, and Semantic Scholar to identify and correct inaccuracies.
3. Galileo
Galileo is a tool that uses external databases and knowledge graphs to verify the factual accuracy of AI outputs. It works in real-time to flag hallucinations and helps developers understand and address the root causes of errors.
4. Cleanlab
Cleanlab focuses on enhancing data quality by identifying and correcting errors in datasets used to train AI models. By ensuring that models are built on reliable information, Cleanlab helps reduce the likelihood of hallucinations.

Best Practices for Responsible AI Use
To use AI tools responsibly and minimize the risk of encountering hallucinated content:
- Cross-Verify Information: Always cross-check AI-generated information with trusted sources.
- Use Fact-Checking Tools: Leverage tools like Factiverse and Galileo to validate content.
- Stay Informed: Keep up-to-date with the latest developments in AI to understand its capabilities and limitations.
- Provide Clear Prompts: When interacting with AI models, use specific and unambiguous prompts to receive more accurate responses.
By understanding the causes of AI hallucinations and utilizing available tools and best practices, users can harness the power of AI responsibly and effectively.
This research highlights the importance of critical thinking and fact-checking when using chatbots. While they can be valuable tools, they are not infallible and can sometimes provide misleading information. As AI technology advances, it’s crucial to understand its limitations and use it responsibly. You should use verification tools to fact-check and use a variety of context analysis methods.
Developers are also working on methods for hallucination reduction in AI models, like implementing feedback loops and increasing training data diversity.